Climate change resilient energy infrastructure through AI-based adaptation (INFRADAPT)
Project duration
Start: 01.04.2024
End: 30.09.2026
Program
Energieforschung 2022
Project Overview
INFRADAPT develops machine learning-based methods for optimal or maximum utilization of existing capacities in low-voltage distribution networks. The impact of climate change on the energy infrastructure and a fair distribution of capacities are considered. The methods are developed and trained for universal use in real time and thus can be used regardless of the network topology. This includes developing and validating (technically and economically) methods for i) optimal placement and sizing of metering infrastructure in low-voltage distribution networks and ii) topology-independent capacity management that allocates available network resources based on metering, AI-based estimation, and AI-based load flow methods.
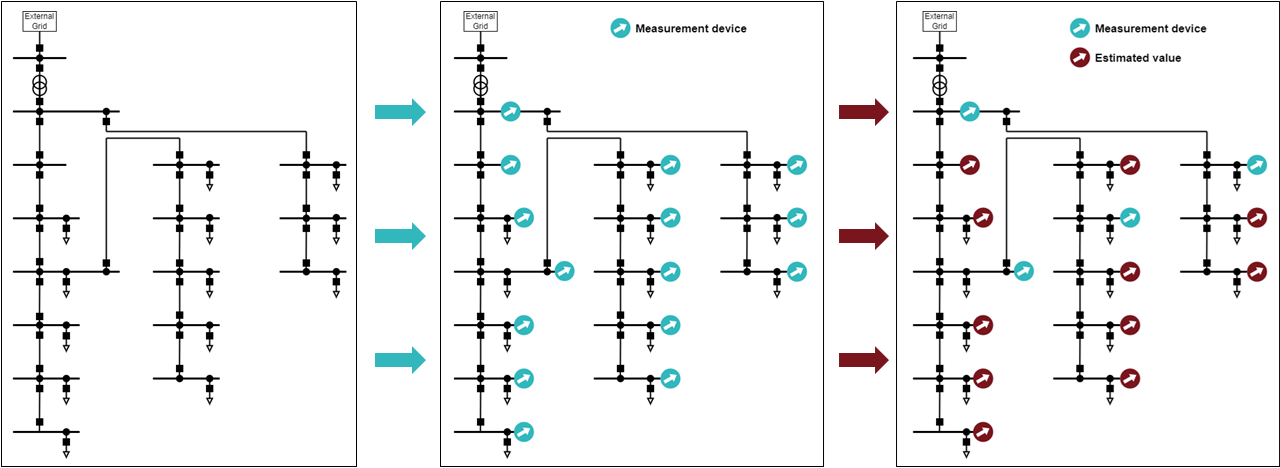
Left: Low-voltage grid completely without measuring infrastructure (problem: no monitoring possible).
Center: Low-voltage grid with complete monitoring (problem: effort, complexity and costs).
Right: Optimal positioning and dimensioning of the measurement infrastructure.
Main Objectives
- Development of methods based on AI for the optimal utilization of grid resources taking into account the effects of climate change
- Optimal placement and dimensioning of the measurement infrastructure in the low-voltage distribution grids to enable a complete and accurate representation of the grid situation together with grid state estimation methods (as part of the subsequent capacity management)
- Topology-independent capacity management (based on measured and estimated values as well as AI-based load flow methods) for optimal and fair distribution of the available grid resources
- Embedding representative climate scenarios: Current grid topology scenarios do not take into account the effects of climate change on renewable energy generation. INFRADAPT analyzes climate scenarios and derives consumption and generation profiles specifically for climate change-related (rare) events as well as for normal operation. The BIFROST [SIE] simulation environment provided by Siemens enables the methods to be validated and the effects on the generation and consumption of various plants to be considered in relation to total energy quantities. Climatic influences create more frequent and extreme situations that have a strong impact on the energy system. Embedding climate scenarios is therefore essential for resilient energy systems of the future.
- Data and communication concept: INFRADAPT is developing a data and communication concept for an efficient and reliable exchange of information to support efficient network operation. In coordination with the methods for capacity management and the measurement infrastructure, a concept for the exchange and transmission of measurement, configuration and control data (time resolution, optimal communication channels, etc.), their processing (decentralized/edge vs. centralized/cloud) and storage is being developed. The aim is to support fault-tolerant and efficient communication between the participants and to identify faulty or manipulated data with the help of AI-based anomaly detection.
- Technical and economic validation: INFRADAPT validates the developed methods with regard to their technical and economic possibilities in compliance with the previously defined key performance indicators. To this end, technical framework conditions (e.g. voltage band, performance limits) and key performance indicators (KPIs; e.g. resilience to erroneous data and outages) are defined and taken into account and continuously reviewed during the development of the methods (iterative development and validation process in WP3, WP4 and WP5) and the relevant stakeholders are involved in the process. General applicability and compliance with grid restrictions are ensured in order to guarantee the best and fairest possible distribution of available grid resources. Economic feasibility is ensured through a cost-benefit analysis, whereby fairness constraints are also taken into account. The aim is to make the methods developed applicable in real time in order to identify and avoid potential grid bottlenecks at an early stage.