Climate change resilient energy infrastructure through AI-based adaptation (INFRADAPT)
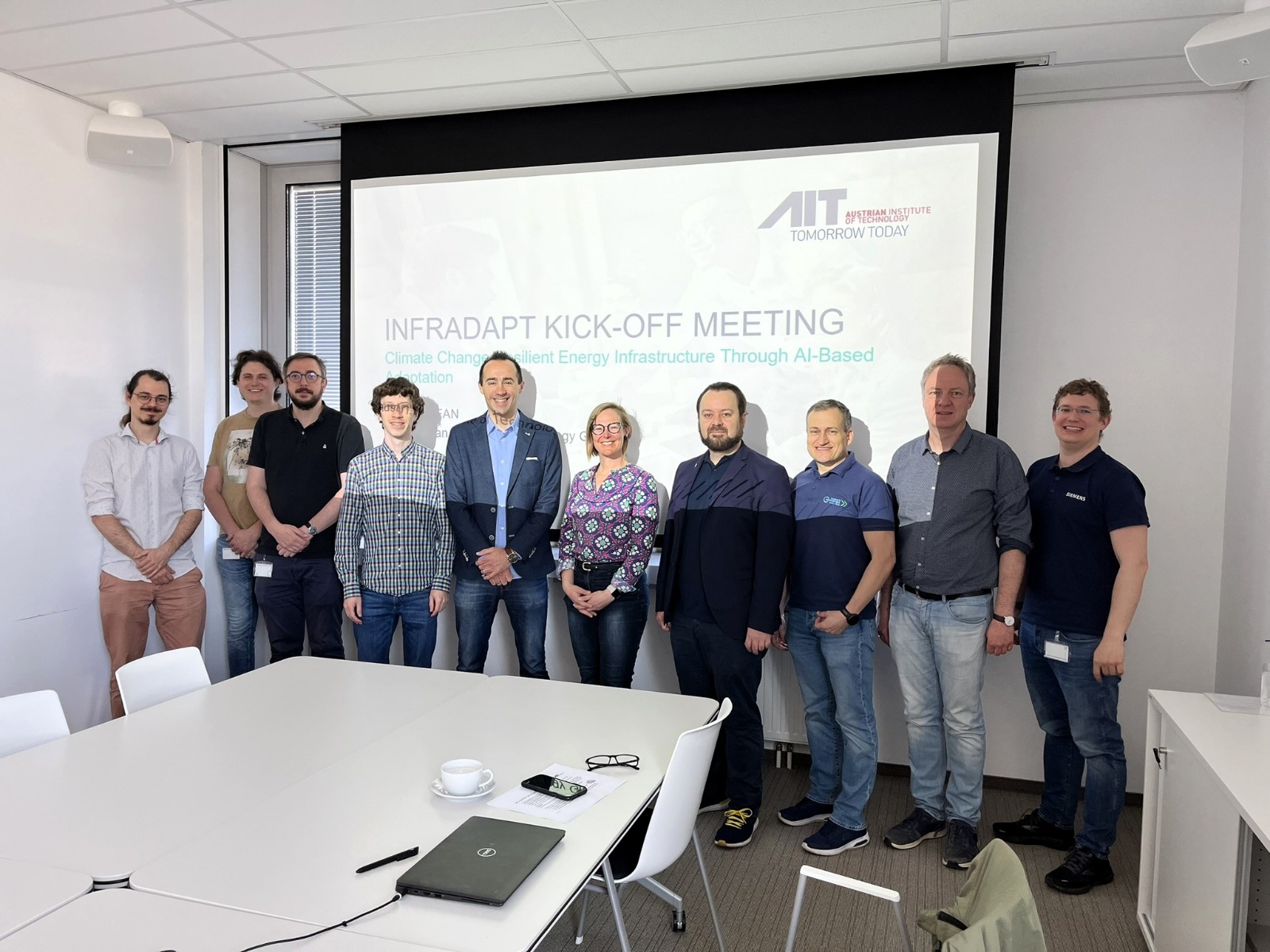
Project Description
The rising temperatures caused by climate change and the increase in cold spells are increasing the demand for electricity for air conditioning and electric heating systems. At the same time, the utilization of infrastructure is increasing due to the electrification of many sectors. Renewable energy sources are essential tools in the fight against climate change. However, their generation capacity is dependent on weather conditions. This leads to constantly growing challenges for the energy infrastructure: high and rapidly changing utilization of operating resources, potential violations of the specified voltage band or deviations in the grid frequency.
Comprehensive monitoring of infrastructure utilization, precise generation and consumption forecasts and knowledge of the flexibility potential of participants are basic prerequisites for maintaining resilient grid operation. However, most low-voltage distribution grids are currently not monitored by metering infrastructure, or only to a limited extent, and smart meter data from customers or local information on generation and consumption as well as relevant physical variables are only available to a limited extent.
Due to the complexity of the system, the high number of participants, their volatility and the large amounts of data, methods from the field of artificial intelligence (AI) in particular represent enormous potential for use in the energy system. They can help to generate additional information for operation and control specifications in real time and thus improve the efficiency and reliability of the system, increase the use of renewable technologies and support the electrification of various sectors.
INFRADAPT develops machine learning-based methods for optimal or maximum utilization of existing capacities in low-voltage distribution networks. The impact of climate change on the energy infrastructure and a fair distribution of capacities are considered. The methods are developed and trained for universal use in real time and thus can be used regardless of the network topology. This includes developing and validating (technically and economically) methods for i) optimal placement and sizing of metering infrastructure in low-voltage distribution networks and ii) topology-independent capacity management that allocates available network resources based on metering, AI-based estimation, and AI-based load flow methods.
Partners
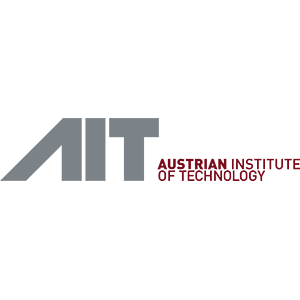
AIT Austrian Institute of Technology GmbH
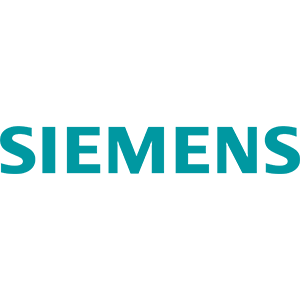
Siemens AG Österreich
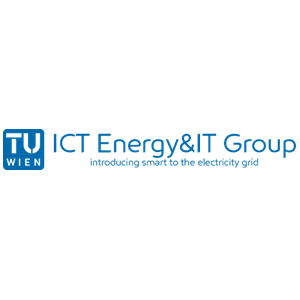
Institute of Computer Technology, TU Wien
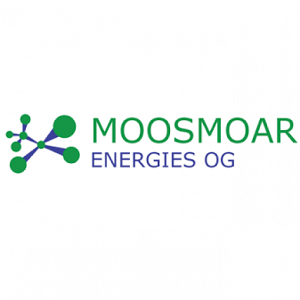